Understanding Explainability and Interpretability in AI: Unlocking the "How" and "Why" Behind AI
Unpacking the implications of Generative AI (Gen AI)
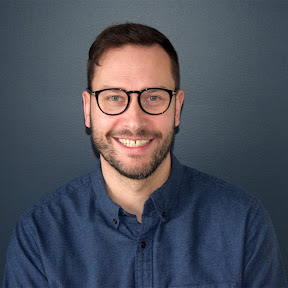
By Francois-Xavier (FX) Jeannet
September 11, 2024
•3 minute read
.jpeg)
This is part of a Geotab series on Responsible AI. It leverages recent work on generative AI, spanning the launch of Geotab Ace and the accompanying Responsible AI Whitepaper as well as our Gen AI Maturity Index.
In today's world, Artificial Intelligence (AI) is becoming an integral part of our daily lives, influencing decisions in areas ranging from healthcare to finance. But as AI systems grow more complex, a crucial question arises: How can we be sure that these systems are making decisions in a fair, transparent, and understandable manner? This is where the principles of explainability and interpretability come into play.
What Are Explainability and Interpretability?
Explainability and interpretability are terms often used interchangeably, but they have distinct meanings:
- Explainability refers to the ability of an AI system to provide understandable reasons for its decisions. It answers the question, "Why did the AI make this decision?"
- Interpretability is about understanding the inner workings of the AI system itself. It answers the question, “How does the AI arrive at its decisions?”
These principles ensure that AI systems are not "black boxes" but transparent entities that can be monitored and understood by humans.
Why Are Explainability and Interpretability Important?
- Trust and Accountability: When AI systems are explainable, users trust them more. For instance, if a driver safety report details why it suggested specific actions, fleet managers are more likely to follow those recommendations. Explainability also supports accountability by clarifying how decisions are made, which is essential for correcting errors and improving the system.
- Ethical and Fair Decision-Making: AI systems can perpetuate biases from their training data. Explainability helps identify and address these biases, ensuring fair and ethical decisions. For example, in hiring, explainable AI can reveal if decisions are influenced by gender or ethnicity, enabling corrective actions.
- Regulatory Compliance: Regulations often require transparency in decision-making. Explainable AI helps organizations comply, avoiding legal issues and maintaining trust. Laws like the EU AI Act and the Colorado AI Act mandate AI governance to manage risks and foster innovation.
- Improved User Experience: Users engage more with AI they can understand. For Geotab Ace, we emphasize explainability by showing how the system works and how it processes queries. The LLM transparently deconstructs user requests, provides the SQL code used, and offers insights into the model’s performance, aiding in troubleshooting and feedback.
How Can We Achieve Explainability and Interpretability?
Designing Transparent Models:
- Some AI models are inherently more interpretable than others. For instance, decision trees and linear regression models provide clear, understandable decision paths, whereas deep neural networks are more complex and less transparent.
- Choosing the right model for the task at hand can enhance explainability without sacrificing performance.
Documentation and Communication:
- Proper documentation (system cards) of the AI system's design, data sources, and decision-making processes is essential. This documentation should be accessible and understandable to non-technical stakeholders.
- Effective communication involves not only documenting the system but also providing tools and interfaces that help users understand the AI's decisions.
Post-Hoc Explainability Techniques:
These techniques are applied after the AI model has made a decision. Examples include:
- Feature Importance: Identifying which features (inputs) were most influential in the decision.
- Local Interpretable Model-Agnostic Explanations (LIME): Creating simpler, interpretable models that approximate the behavior of complex models for specific decisions.
- SHapley Additive exPlanations (SHAP): Using game theory to explain the contribution of each feature to the final decision.
What is Unique About Generative AI in Terms of Explainability?
While generative AI opens up exciting new possibilities, it also introduces unique challenges in terms of explainability:
Complexity and Scale:
- Generative AI models are often based on deep neural networks with millions or even billions of parameters. This sheer complexity makes it difficult to trace how specific inputs lead to specific outputs.
- Unlike simpler models, where you can follow a clear decision path, generative models involve intricate layers of computation that are not easily interpretable.
Creative Outputs:
- Generative AI is designed to produce creative and novel outputs, which means its "decisions" are not just about classification or prediction but about generating entirely new content. Explaining why a model generated a particular piece of text or image is inherently more challenging than explaining a classification decision.
- The subjective nature of creativity adds another layer of complexity. What constitutes a "good" or "appropriate" output can vary widely depending on context and user expectations.
Context Sensitivity:
- Generative AI models are highly sensitive to context. Small changes in the input can lead to significantly different outputs. Explaining these variations requires a deep understanding of how the model interprets and processes context.
- For example, a generative text model might produce different responses based on subtle nuances in the input prompt, making it challenging to provide a clear rationale for each output.
Training Data Influence:
- The outputs of generative AI are heavily influenced by the data they were trained on. Understanding and explaining the biases and patterns in this training data is crucial for interpreting the model's behavior.
- Transparency about the training data and the methods used to curate and preprocess it becomes essential for explainability.
Ethical Considerations:
- Generative AI can inadvertently generate harmful or biased content. Explaining how and why such content was produced is vital for addressing ethical concerns and improving the model.
- Ensuring that generative models adhere to ethical guidelines requires robust explainability mechanisms to identify and mitigate potential risks.
As AI evolves, explainability and interpretability are key to ensuring transparency, trust, and fairness. Designing AI to explain its decisions enhances performance, upholds ethical standards, and fosters responsible integration into society. For generative AI, addressing these aspects is crucial for balancing creativity with transparency and accountability.
Subscribe to get industry tips and insights
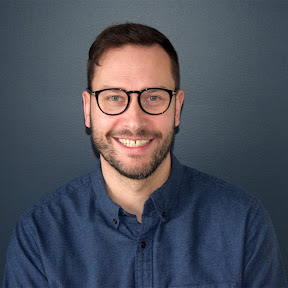
Cyber Risk Advisor and Data & Technology Enthusiast with over a decade of experience delivering transformative projects in the financial services and insurance industries. As a respected privacy and AI risks advisor, FX leads the development of comprehensive action plans to address privacy risks in the AI era. With a strong background in privacy, data protection, and IT/IS business solutions, FX is also passionate about fintech, insurtech, and cleantech. He combines his technical expertise with excellent communication and relationship management skills to guide his business partners to success.
Table of Contents
Subscribe to get industry tips and insights
Related posts
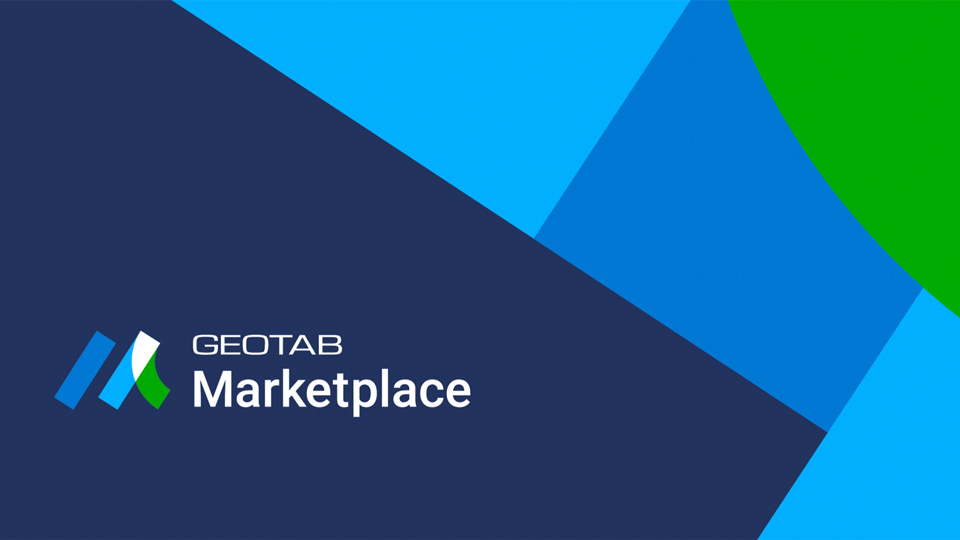
Marketplace Spotlight: Behind the Vision of Predictive Coach
January 30, 2025
1 minute read
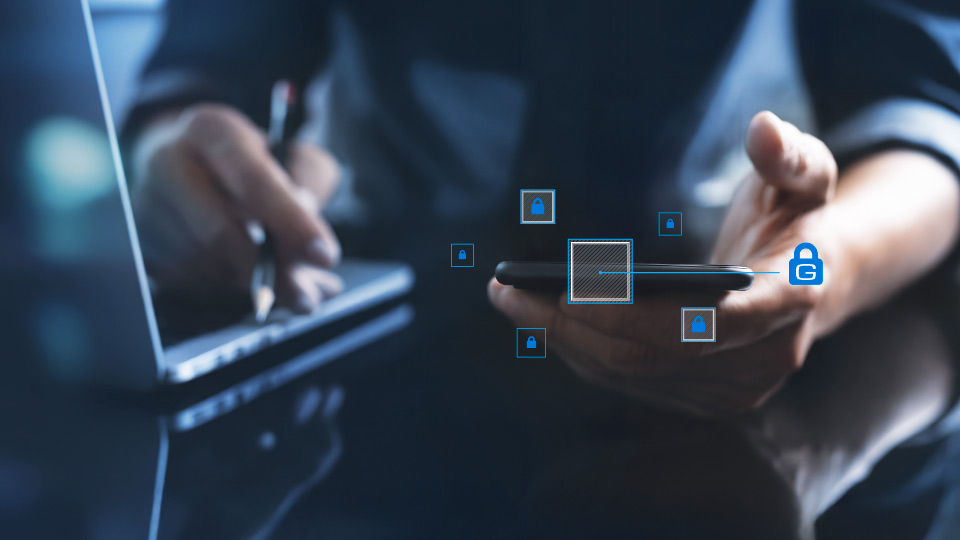
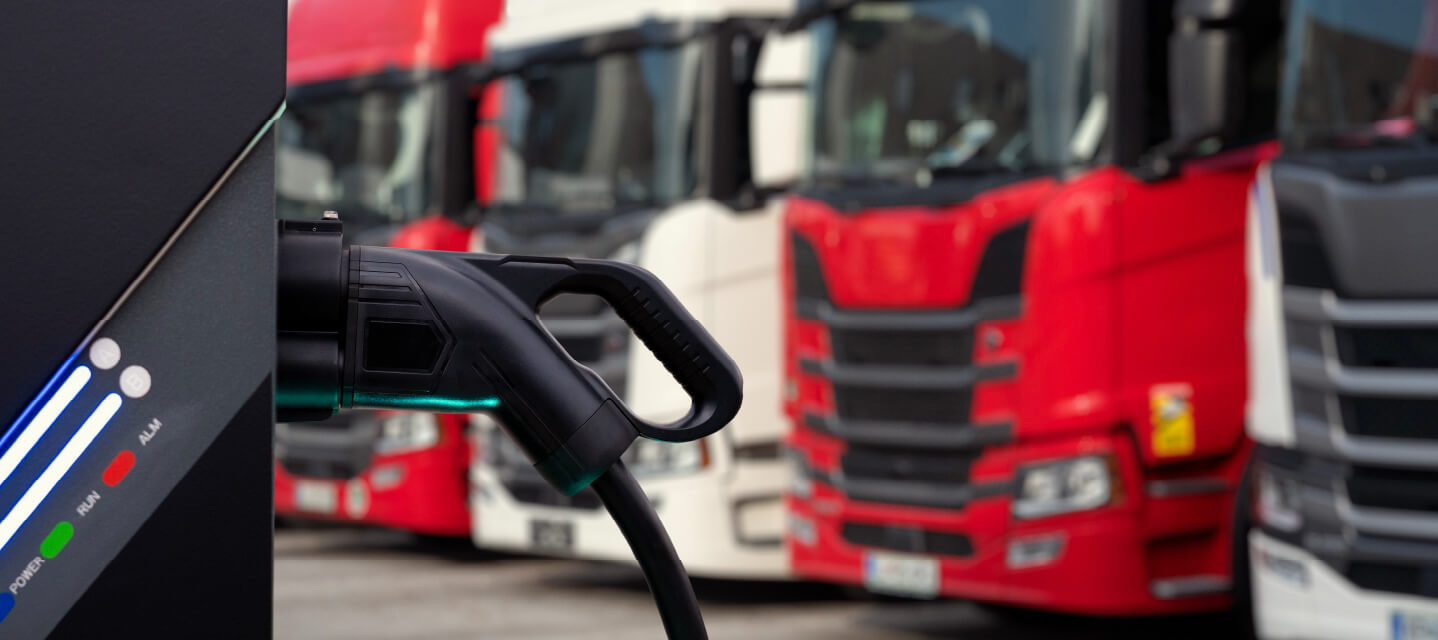
How long do electric car batteries last? What analyzing 10,000 EVs tells us…
January 20, 2025
9 minute read
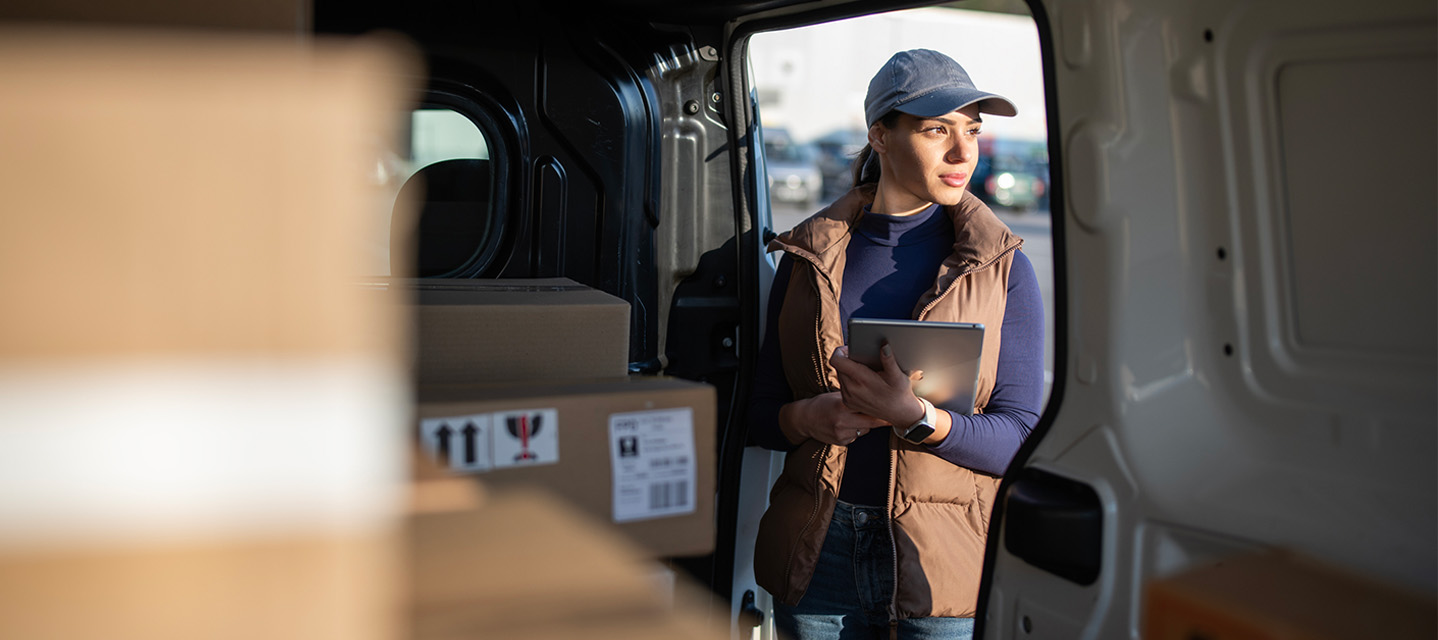
How last mile delivery fleets are customizing the Geotab solution
January 17, 2025
5 minute read
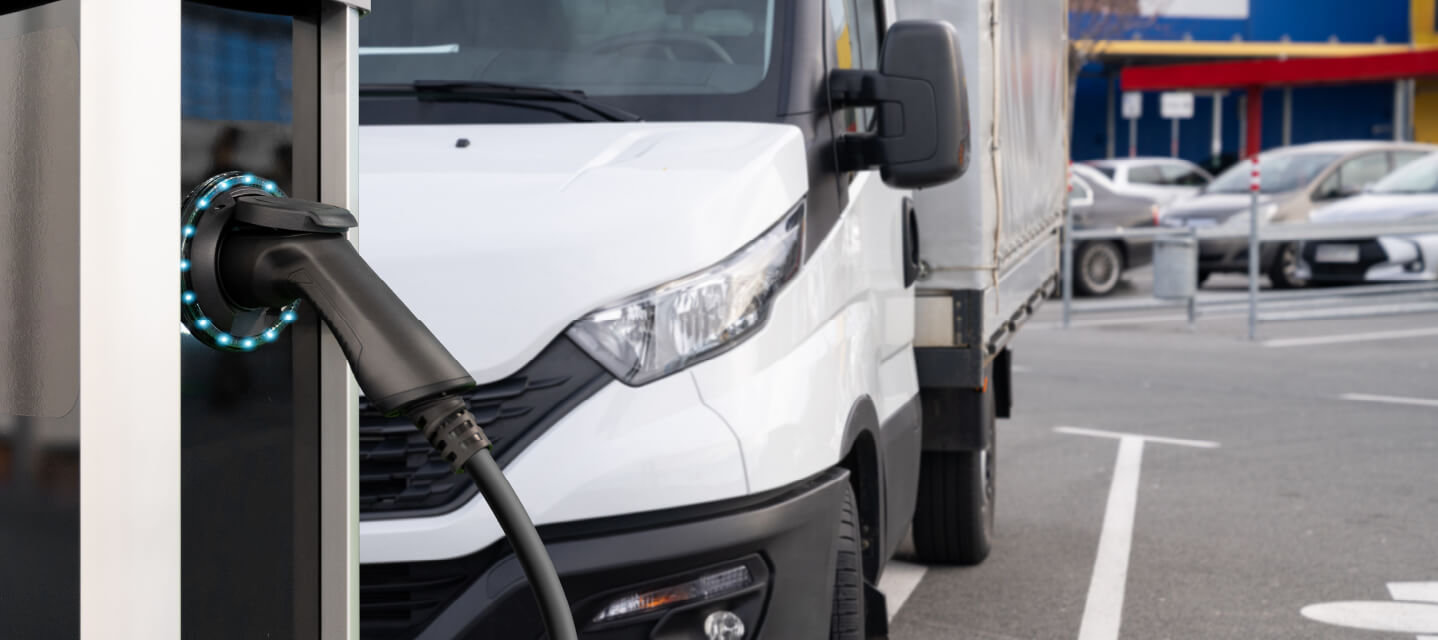
What is fleet electrification? (+ How to build a sustainable strategy)
January 13, 2025
4 minute read
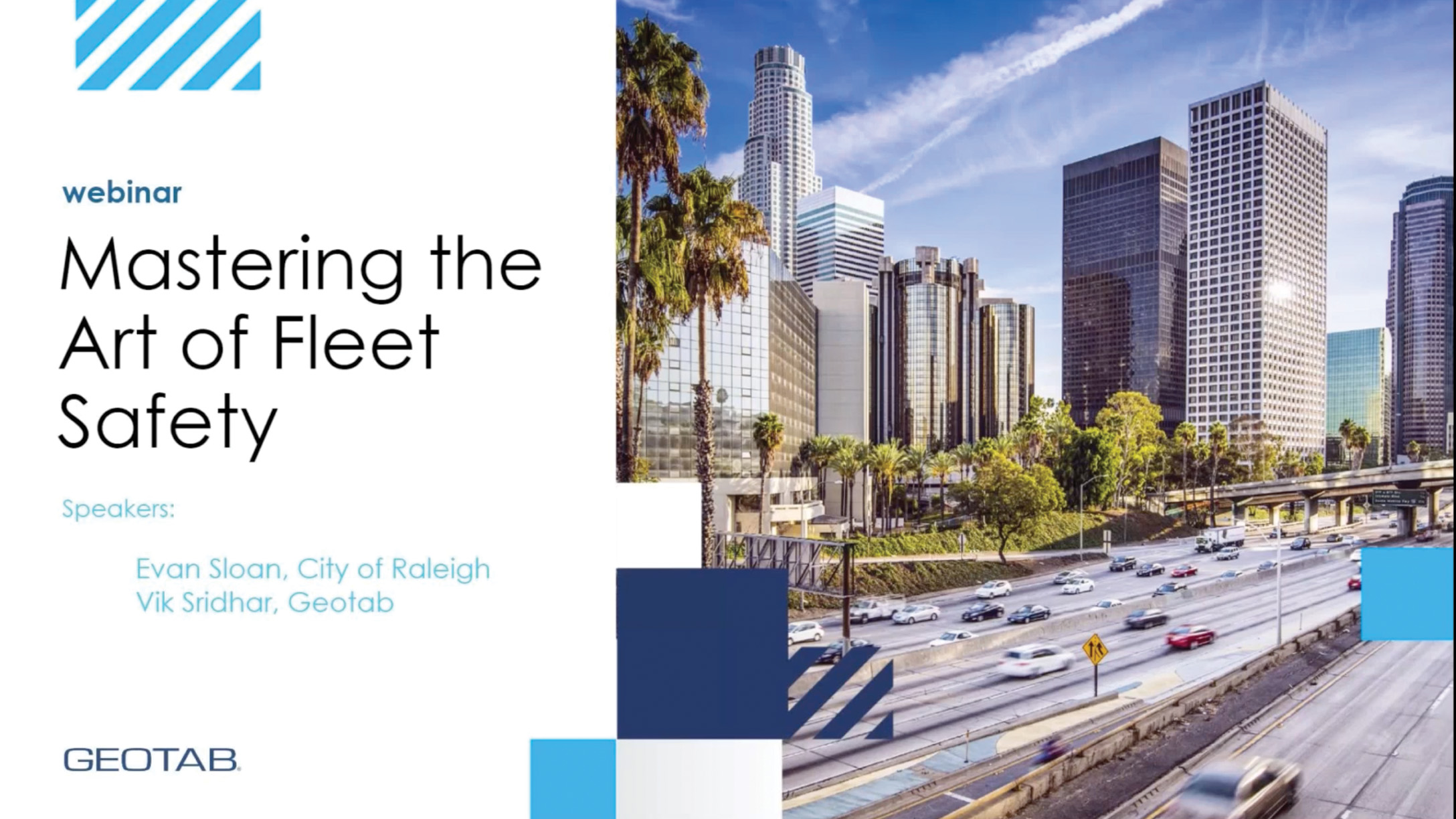
Developing a next-gen government fleet safety program: Made possible with AI
November 26, 2024
2 minute read