Predicting traffic congestion with driving behavior
Does your fleet spend too much time stuck in traffic? A big data developer discusses traffic congestion prediction and how Geotab technology can help.
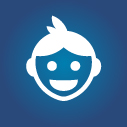
By Geotab Team
Published: Dec 7, 2015
•7 minute read
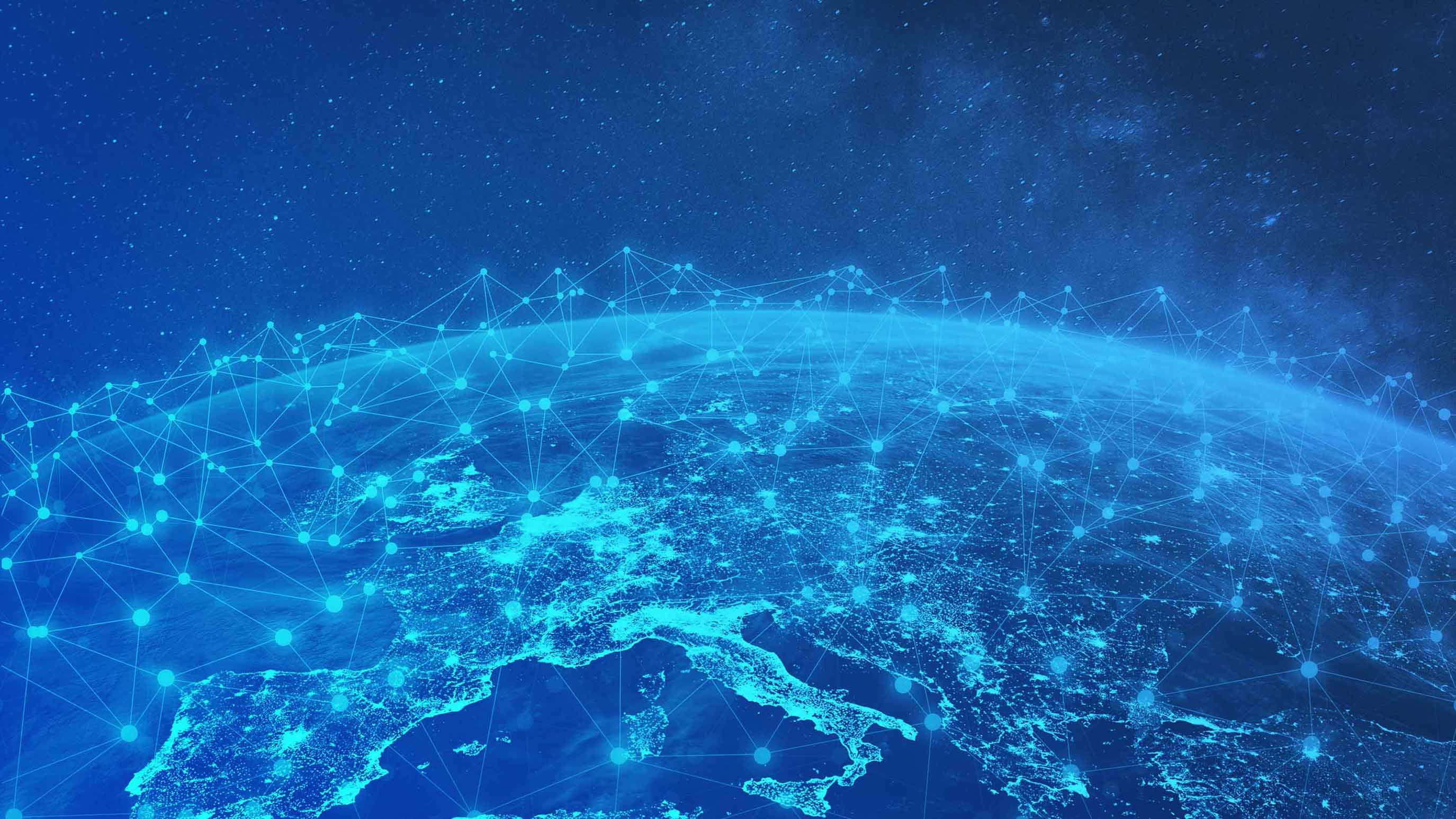
Traffic congestion imposes a significant economic impact on industrialized society. How many hours a week do your drivers spend stuck in traffic?
The prediction of traffic congestion has been actively researched as one of the most effective solutions, to support proactive traffic management and planning. This article provides an overview of traffic congestion and the challenges of tackling congestion through different models of prediction.
See also:
Can you predict wait times at major city intersections?
Simplifying data exploration and visualization with Geotab Ignition
Background: The negative impact of traffic congestion
Traffic congestion is a problem that began more than a century ago when the introduction of the assembly line in the automotive industry made it possible to mass-produce automobiles. The problem of traffic congestion has not yet been resolved and still causes countless losses in most industrial countries.
The negative effects of traffic congestion:
- Congestion decreases the traffic volume that can use the roadway.
- Delays due to congestion cost Americans $121 billion in the form of 5.5 billion lost hours and 2.9 billion gallons of wasted fuel in the USA alone. [1]
- Congestion makes it hard to estimate traffic time. As shown in Figure 1, in the USA, people have to spend a surprising amount of time on trip planning due to congestion.
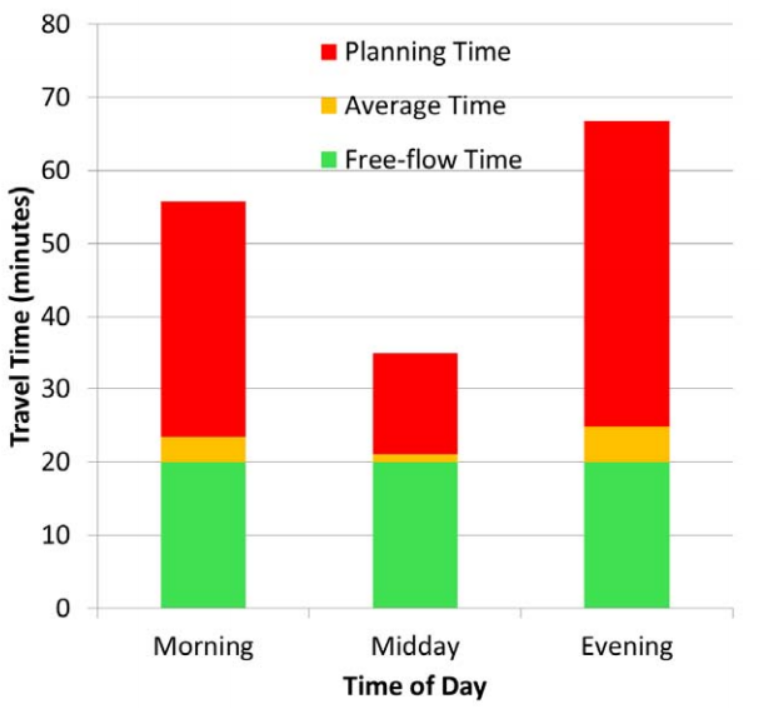
Figure 1. Extra time to make important trips.[1]
Ways to reduce congestion
Three methods of reducing congestion are commonly considered [2]:
- Increase transportation capacity by constructing more freeways. This solution considers road saturation as a natural way to explain traffic congestions. The demand for space on the roads is greater than what is available. However, research uncovered a phenomenon called generated traffic [18] – i.e. increasing road capacity can even stimulate more demands than newly increased capacity can take, thus may lead to even worse traffic. Moreover, further construction of freeways to alleviate congestion is unlikely in many cities today.
- Promoting public transport in large cities. While this may be a good solution, it is not always convenient for people to use.
- Employ proactive traffic management and planning. Under such circumstances, proactive traffic management and planning to improve traffic efficiency is considered to be a major way of alleviating traffic congestion. This requires determining the future state of road segments, i.e. predict potential congestions before real congestion is reached.
Proactive congestion detection and prediction
Traffic dynamic models are the foundation of predictions. Both macro and micro levels of traffic dynamic has been intensively studied. Macroscopic dynamic models are good at explaining recurring traffic congestion patterns on a large-scale network perspective while microscopic dynamic models explore individual vehicle behaviors and are very effective in stimulating the instabilities of traffic waves and non-recurring congestions caused by unpredictable incidents.
Despite the popularity of traffic dynamic models, not so many practical methods of congestion prediction have been developed and deployed, especially for non-recurring congestions which are responsible for more than half of congestion cost. Based on literature reviews, this article discusses a novel method of real-time, short-term non-recurring congestion prediction, which integrates individual vehicle behavior data collected by telematics technologies and research results of modern traffic dynamic models.
Proactive congestion detection and prediction was virtually an untouched area before Kaysi et al. [3] set up a framework for a real-time Advanced Traveller Information System (ATIS). Real-time diversion of traffic is expected to be implemented through this system. In brief, wireless communication infrastructures and navigation technologies are exploited to:
- Collect real-time data from surveillance system,
- Infer relevant information concerning congestion, and
- Provide congestion information through a control and routing (CAR) module to each single driver. Congestion prediction is critical for the effectiveness of ATIS.
The challenges of congestion prediction are rooted in the complex traffic flow dynamic. Real-world traffic flow is a multi-loop, multi-state, non-linear feedback system that reacts to the individual decision maker’s or driver’s actions in both anticipated and unanticipated ways. Traffic dynamic has been intensively studied since 1950’s. Two perspectives are widely used to simulate traffic dynamic: the microscopic view and macroscopic view.
- Macroscopic traffic flow simulates the behaviors of the traffic stream overall (road density, vehicle velocity and volume).
- Microscopic traffic flow simulates the behaviors of individual vehicles.
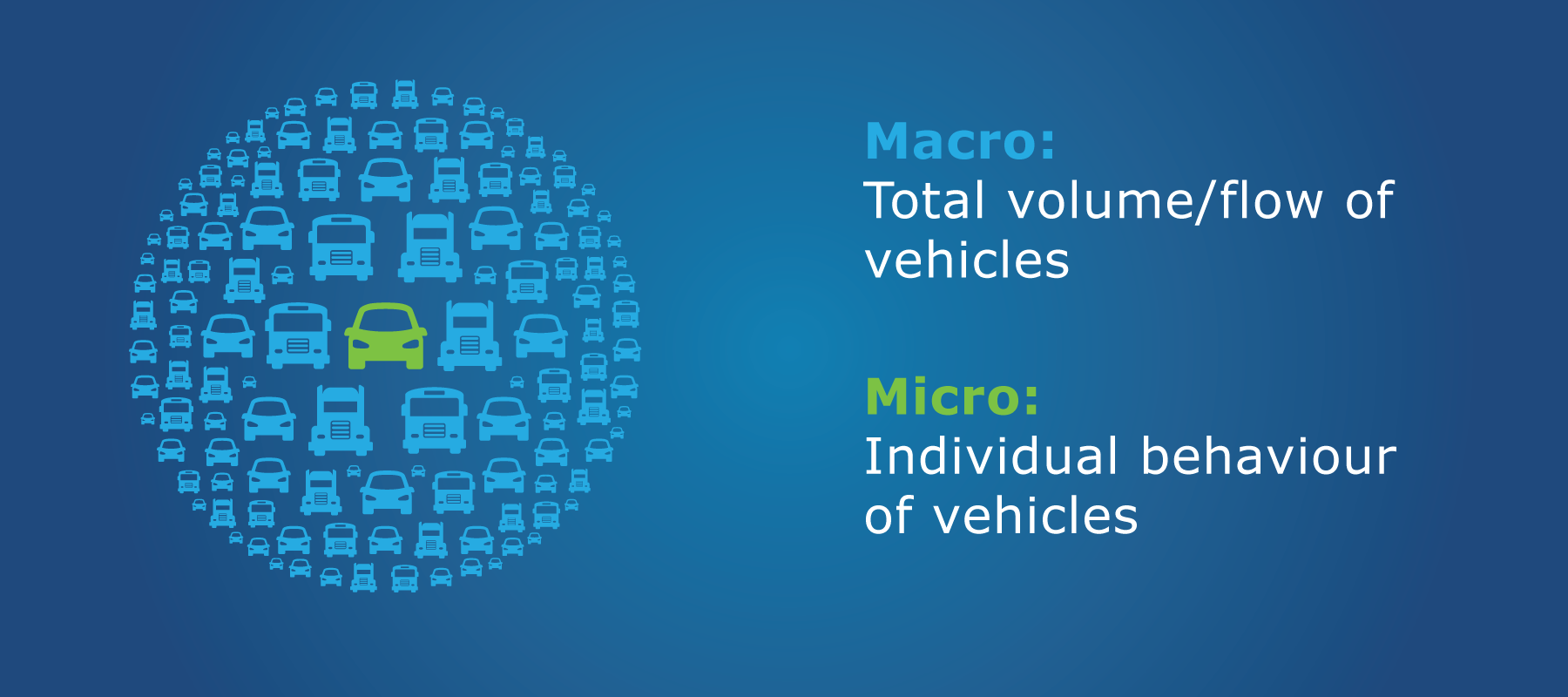
Figure 2. Macroscopic versus microscopic.
Different models have been developed to explain congestion based on these two perspectives.
Macroscopic models are quite effective in explaining recurring congestion. This type of congestion reflects the day-to-day build-up of traffic on urban expressways and arterials, notably during the morning and afternoon commuter peak periods. The regularity of this congestion allows its measurement relatively straightforward. Common measurements include macroscopic features such as traverse time, average speed and density.
Macroscopic models have been intensively researched and a few of them have been implemented in real traffic management systems. They helped to discover recurrent congestion patterns of large scale traffic networks in question. However, macroscopic models are less sensitive to another type of congestion, non-recurring congestion, which is responsible for two-thirds of traffic delays [1,7].
Non-recurring congestion is caused by roadway incidents or other unexpected events that temporarily disrupt the flow of traffic on a segment of a roadway.
When an incident occurs, there is a temporary decrease in the capacity of the roadway and if current demand exceeds this reduced capacity it results in congestion, thus forming queues.
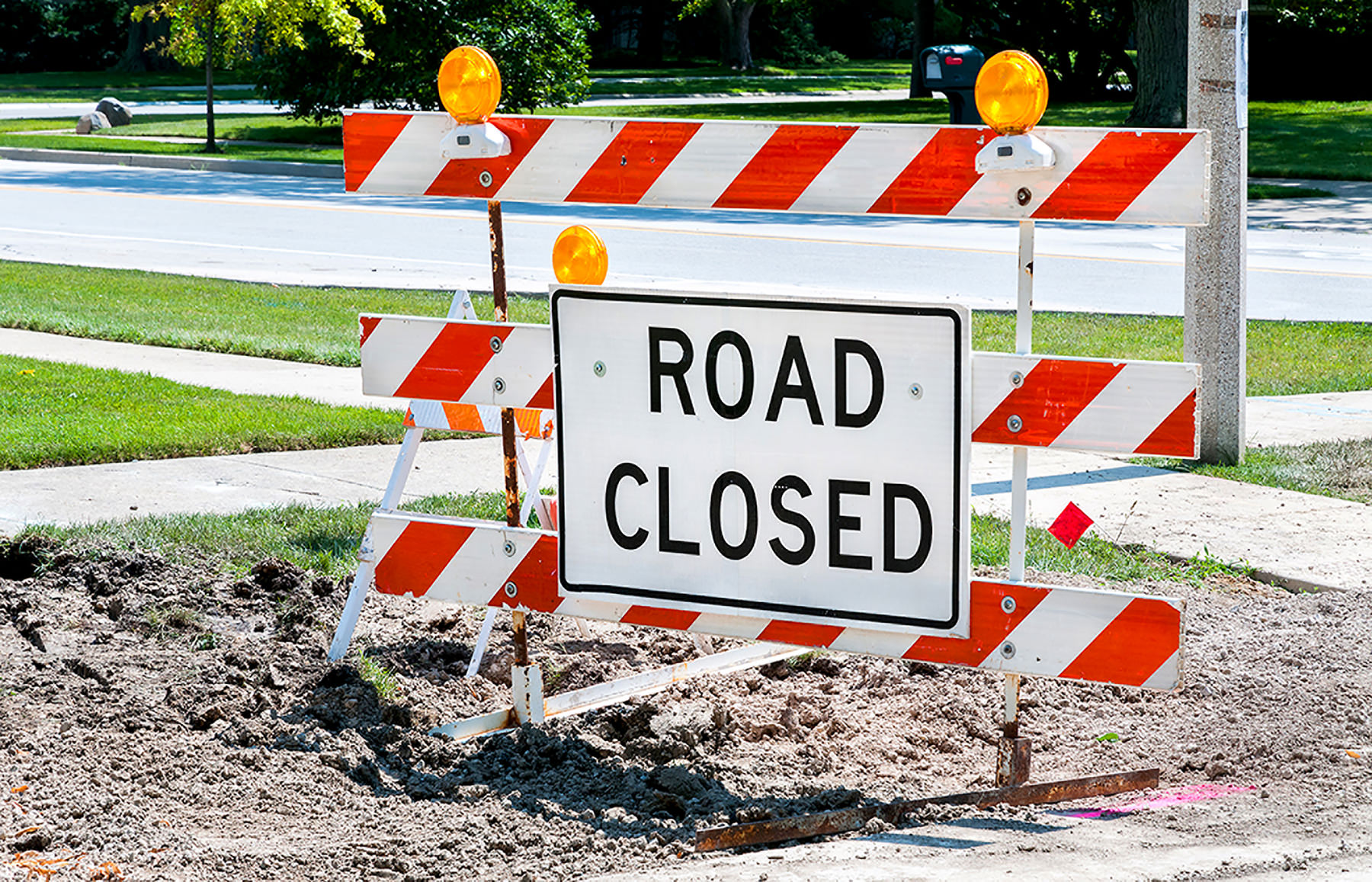
Figure 3. Non-recurring congestion such as work zones temporarily disrupt traffic flow.
When vehicle density is large enough (~30/km), a random event such as a fluctuation in a leading car’s velocity can induce a transition from free flow to jam. This explains the phenomenon where for no apparent reason, traffic will slow to a crawl or even a halt and after several minutes return to normal speeds. Such stop-and-go roads only carry ½ to ⅔ of the vehicles as a smoothly flowing road. Macroscopic aggregate data is less straightforward to fit in the explanations. [4]
Due to complex human factors, it is only in recent years that microscopic models have been well established to simulate non-recurring congestion phenomenons. Mathematicians from the University of Exeter developed a math model [6] revealing that by slowing down below a critical speed when reacting to such an event, a driver would force the car behind to slow down further and the next car back to reduce its speed further still. The result of this is that several miles back, cars would finally grind to a halt. Flynn et al. [5] coined the term phantom traffic jams to describe jams that arise in the absence of any obstacles.
They found that the fundamental features of traffic waves (jamitons) in phantom traffic jams are similar to detonation waves produced by explosions and argued that the exact shape and the speed of propagation of jamitons could be predicted.
However, no practical prediction methods have yet been developed to apply to non-recurring congestions. A fundamental challenge is the general lack of data at microscopic levels for incident detection. [7]
How vehicle telematics helps drivers manage traffic congestion
Vehicle Telematics has been defined as the convergence of telecommunications, information processing and vehicle technologies and road transportation [8]. GPS fleet management and vehicle tracking can play an important role in handling traffic congestion. For example, vehicles equipped with Geotab technology have successfully realized safe trips and significant cost savings benefits. Figure 4 illustrates a high-level architecture of the Geotab fleet management system.
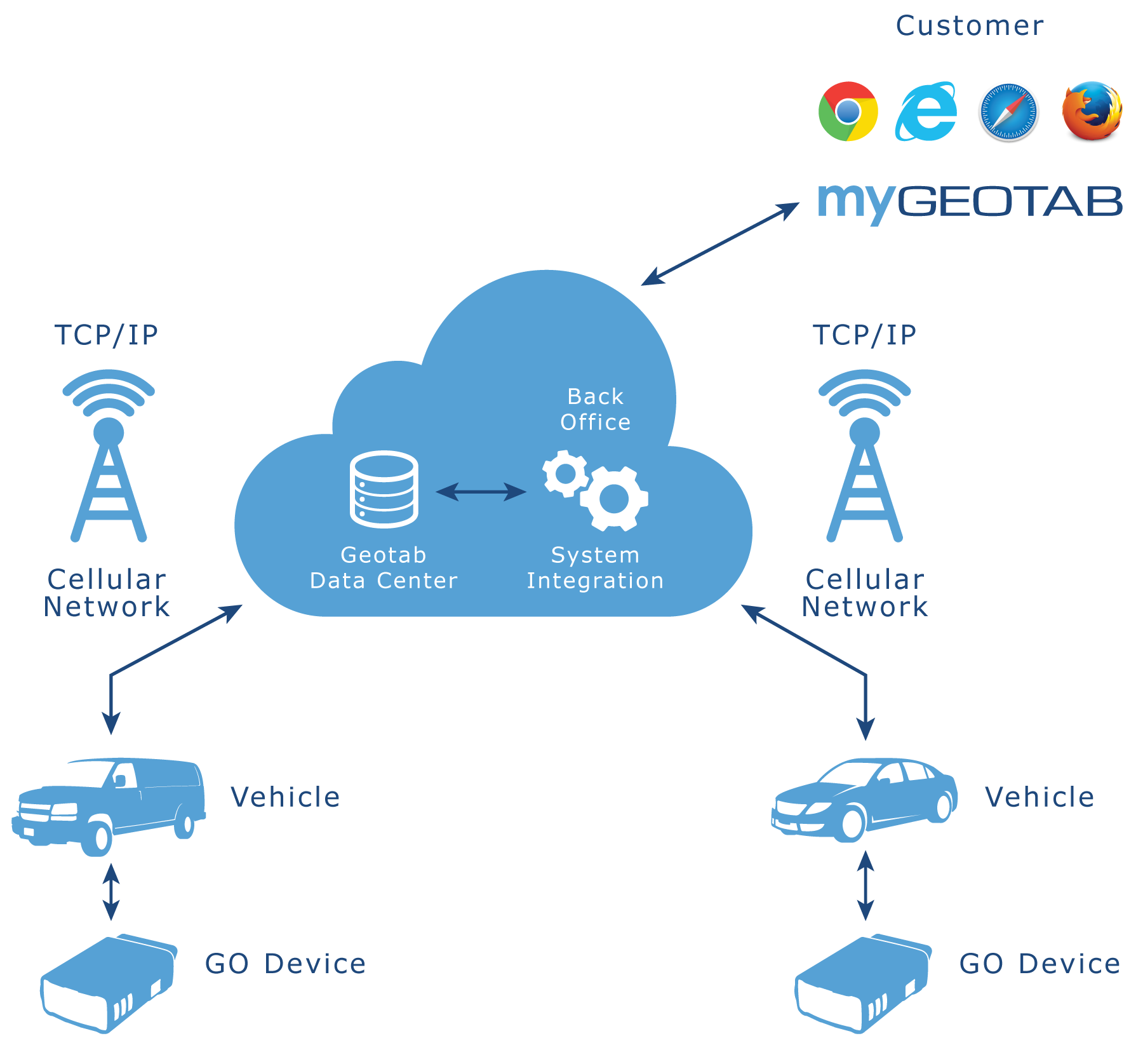
Figure 4. A high-level picture of the architecture of Geotab fleet management system.
In this architecture, each vehicle (referred as “Geotab probe vehicle” in the following) has one Geotab GO device plugged into the on-board diagnostics (OBD) interface. After several minutes of warm-up at the first time of being installed, the GO device starts to retrieve real-time OBD information, such as:
- Engine status
- Fuel level
- Mileage
- Speed
- Seat belt usage status
- Time when brake is peddled
The GO device is also embedded with a GPS chip and an accelerometer to detect host vehicle’s location and acceleration. In every second, over 10,000 of such real-time track records are transferred upward to Geotab servers for further processing. Derived info around driving behaviors, such as unexpected harsh speeding or decelerating, turning radius and idling without ignition off, as well as vehicle model specific features, such as inertia and fuel consumption, are calculated. As of now, Geotab allows its end users to view this information in real-time within Geotab’s web-hosted reporting environment and in turn achieve smarter fleet management. GO devices are also capable of transferring downward route plan instruction from fleet managers to matched Garmin Navigation System [9] on host vehicles.
Geotab fleet management architecture unintentionally conforms with ATIS framework.
With Geotab technology, comprehensive and detailed info around individual vehicle behaviors become available and measurable.
This triggers a great applied research interest in exploring the viability of utilizing data from Geotab devices in real-time, short-term (i.e. 5-30 minutes) congestion predictions, i.e. when and where congestion is likely to occur on the freeway network. Comparing to predictions purely based on macroscopic traffic features, deeply exploring and properly utilizing individual behavior data has advantages in identifying anomalies which leads to stop-and-go traffic and in turn predict congestions at the very early stage. Such work is also expected to put valuable insights into the current Geotab vehicle trip planner and future automatic fleet control system.
Does your fleet spend too much time stuck in traffic? Learn more about Geotab’s proactive solutions for fleet management, or contact us to find the Geotab solution for your needs.
References:
- Schrank, D., Eisele, B. & Lomax, T. (2012, December) TTI’s 2012 Urban Mobility Report. Retrieved from http://media2.kjrh.com/html/pdfs/2012urbanmobilityreport.pdf
- Lécué, F., Tucker, R., Bicer, V., Tommasi, P., Tallevi-Diotallevi, S., & Sbodio, M. (2014). Predicting Severity of Road Traffic Congestion Using Semantic Web Technologies. In The Semantic Web: Trends and Challenges (pp. 611-627). Springer International Publishing.
- Kaysi, I., Ben-Akiva, M., & Koutsopoulos, H. (1993). Integrated approach to vehicle routing and congestion prediction for real-time driver guidance (No. 1408).
- Mangharam, R., Lee, I., & Sokolsky, O. (2008). Real-Time Traffic Congestion Prediction.
- Flynn, M. R., Kasimov, A. R., Nave, J. C., Rosales, R. R., & Seibold, B. (2008). On “jamitons,” self-sustained nonlinear traffic waves. arXiv preprint arXiv:0809.2828.
- Traffic Jam Mystery Solved by Mathematicians. (2007, December 19). Retrieved from http://phys.org/news117283969.html
- iTRANS Consulting Inc. Costs of Non-Recurrent Congestion in Canada Final Report. (2006, December 11). Retrieved from http://www.bv.transports.gouv.qc.ca/
- Telematics (n.d.) In Wikipedia. Retrieved from http://en.wikipedia.org/wiki/Telematics
- Garmin Navigation System. http://www.garmin.com/
- Huisken, G. (2006). Inter-urban short-term traffic congestion prediction. University of Twente.
- Nihan, N. L. (1995). Freeway congestion prediction (No. WA-RD 381.1). Washington State Department of Transportation.
- Marfia, G., Roccetti, M., & Amoroso, A. (2013). A new traffic congestion prediction model for advanced traveler information and management systems.Wireless Communications and Mobile Computing, 13(3), 266-276.
- IBM Traffic Prediction Tool. http://researcher.watson.ibm.com/researcher/view_group_subpage.php?id=1248
- Orosz, G., Wilson, R. E., & Stépán, G. (2010). Traffic jams: dynamics and control. Philosophical Transactions of the Royal Society A: Mathematical, Physical and Engineering Sciences, 368(1928), 4455-4479.
- Kometani, E. & Sasaki, T. (1958) On the stability of traffic flow. J. Oper. Res. Soc. Japan. 2, 11–26.
- Newell, G. F. (2002). A simplified car-following theory: a lower order model.Transportation Research Part B: Methodological, 36(3), 195-205. Orosz et al. 2009.
- Orosz, G., Wilson, R. E., Szalai, R., & Stépán, G. (2009). Exciting traffic jams: Nonlinear phenomena behind traffic jam formation on highways. Physical Review E, 80(4), 046205.
- Litman, T. (2001). Generated traffic and induced travel. Victoria Transport Policy Institute, 22.
Subscribe to get industry tips and insights
Updated: Dec 27, 2023
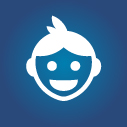
The Geotab Team write about company news.
Table of Contents
Subscribe to get industry tips and insights
Related posts
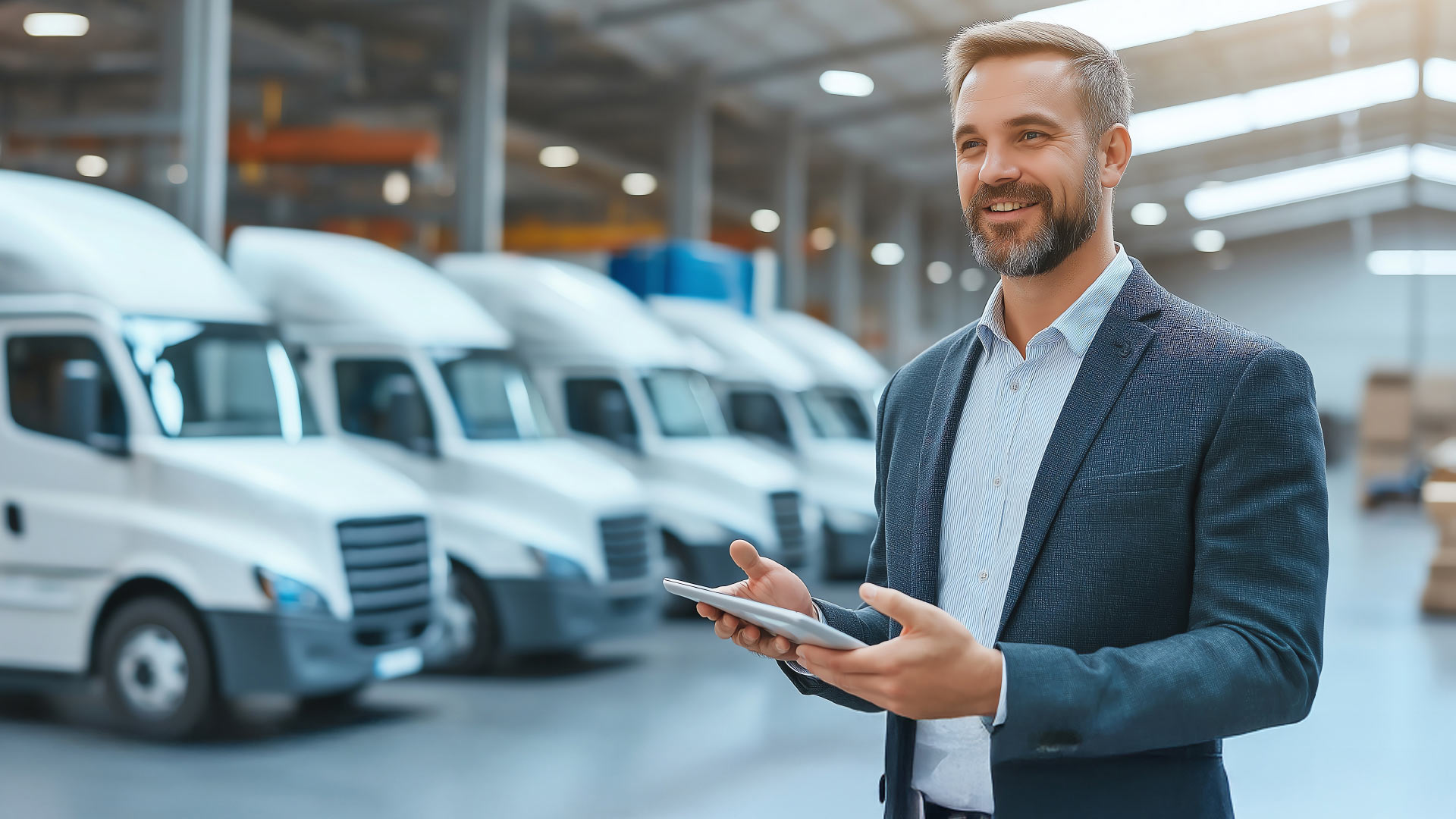
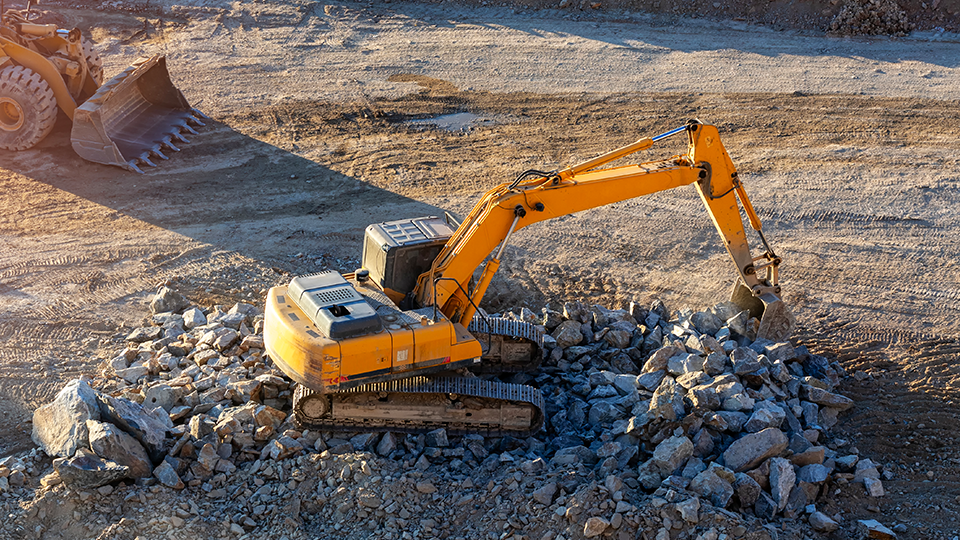
How to avoid high downtime costs for construction fleets
March 10, 2025
2 minute read
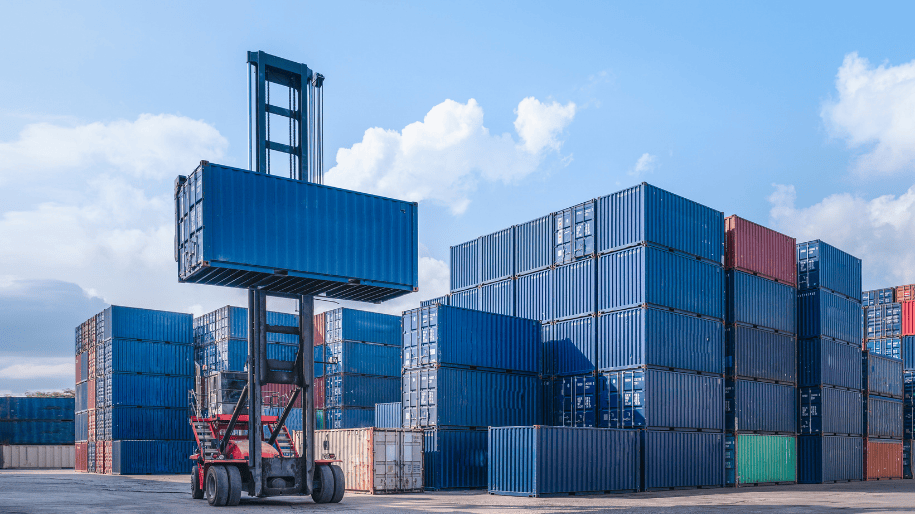
Track anything, anywhere, with the GO Anywhere asset tracker
March 6, 2025
3 minute read
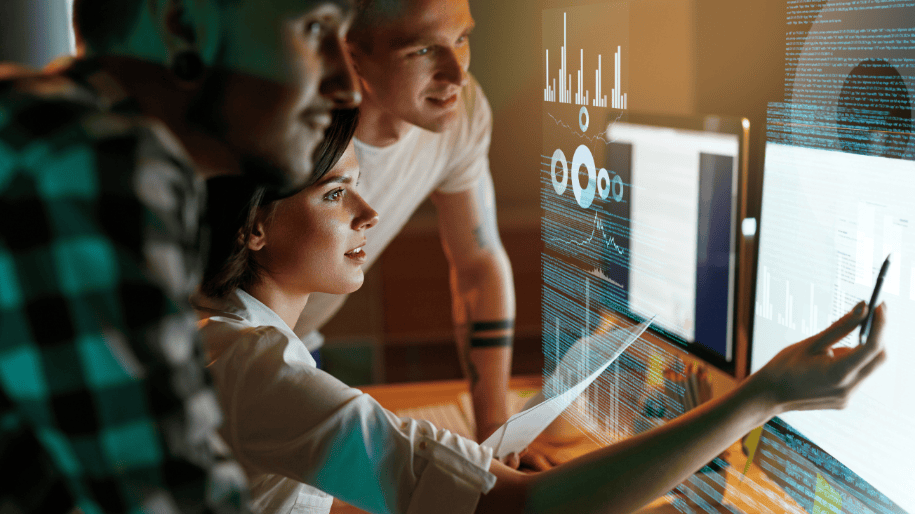
Geotab Data Connector gets an upgrade for better fleet insights
February 24, 2025
1 minute read
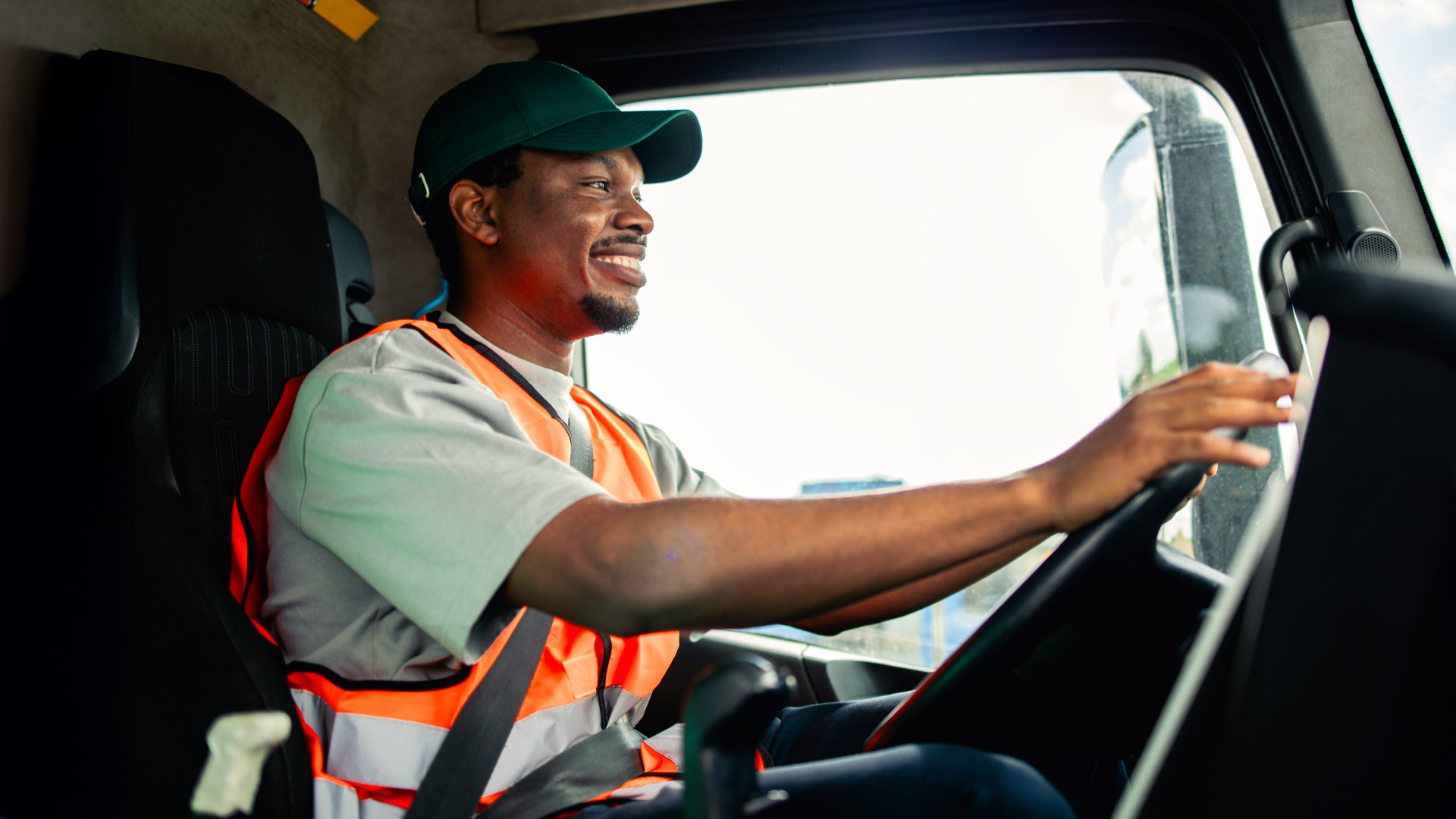
Driver incentive programs to improve safety, retention and fleet efficiency
February 7, 2025
4 minute read
.jpg)
School bus fleet management 101: A comprehensive guide
February 5, 2025
4 minute read